AI is omnipresent in financial services. That is at least the conclusion of the NVIDIA State of AI in Financial Services 2024 Report, in which NVIDIA outlined the key AI trends for financial institutions today and moving forward. Based on over 500 interviews with executives from banks, investment firms and insurance companies worldwide, NVIDIA concluded that 91 percent of financial institutions are either already assessing AI or deploying AI solutions in production. As reported, AI use cases range from operations over risk & compliance to sales. The advantages of AI solutions are ubiquitous: banks can seriously boost their operational efficiency through automated AI decision-making and employ systems that continuously learn by algorithmically adapting to new data patterns rather than exclusively relying on manual updates.
“A naïve application of AI systems is prone to many drawbacks.”
While in 2024 and beyond, AI is continuing to be on the rise, there is one problem. A naïve application of AI systems is prone to many drawbacks. Especially in high-risk use cases within financial services, these can have direct and severe impacts on the fundamental rights of individuals. Take the example of automated credit risk scoring and the danger of systematically mistreating members from particular ethnic groups. It goes without saying that an AI model would seldom have direct access to sensitive information like ethnicity when evaluating an applicant’s creditworthiness.
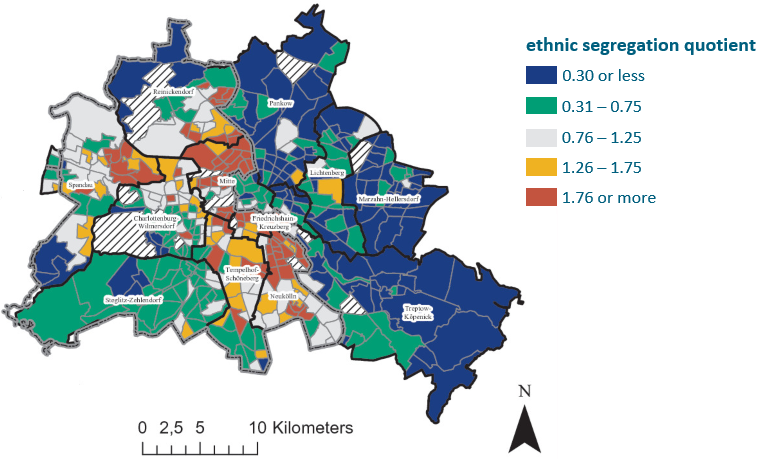
The problem is that AI models might very well base their decision-making at least partly on information that implicitly contains ethnic information without this ever being intended during model development.
“If you are training a machine learning model with the goal of not discriminating against different ethnicities while including geo-spatial data into the model, you failed.”
To illustrate this point, let us focus on a study about spatial segregation of different demographic groups published in 2021 by Talja Blokland and Robert Vief. As the authors show in the figure presented above, even within one city, members of different ethnicities heavily cluster geographically across different city districts. A data science development team might be training a machine learning model with the goal of not discriminating against different ethnicities. But: if the model is granted access to geo-spatial information like properties of applicants’ city districts or zip codes into the model, the model is essentially picking up ethnic cues. As visualized in the figure, such a model would not refrain from using ethnicity as a feature for prediction. It would simply use a more latent representation of ethnicity – as hiddenly encoded in geo-spatial information.
As a consequence, individuals who are similar in all other attributes (for instance income, age, etc.) might suffer a systematic unequal treatment simply due to their membership in different ethnic groups, as visualized in the graphic below.
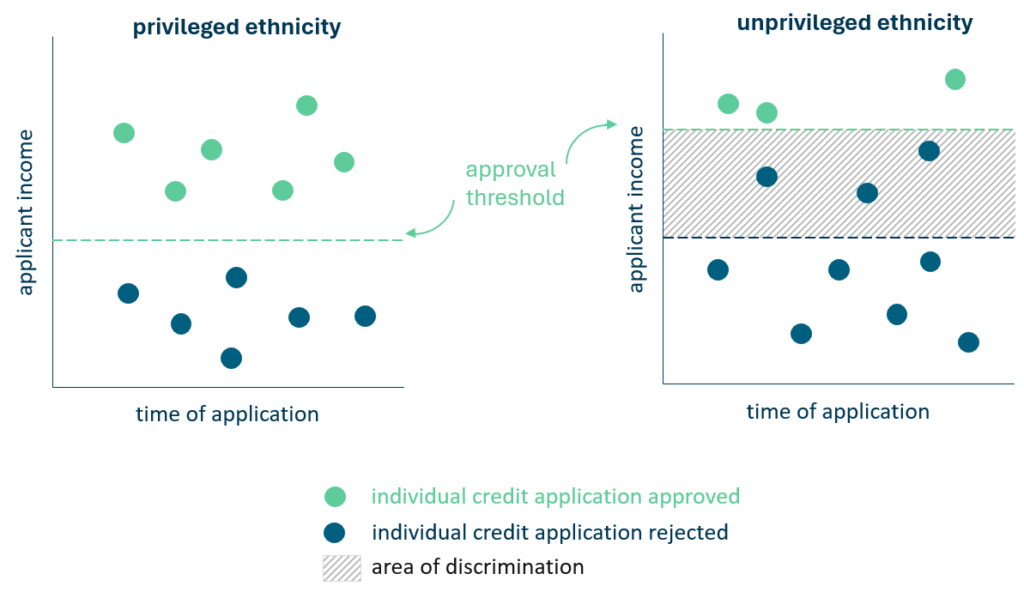
“Responsible AI has many dimensions – and they are getting regulated”
Of course, machine learning fairness is by far not the only characteristic by which AI systems need to be made responsible and ethically sound. Earlier this year, the European Parliament officially adopted the EU Artificial Intelligence Act – the first encompassing international legislation on the use of AI systems within the European market. Especially for high-risk use cases, the new legislation comes with vast regulatory requirements. Coming into force on August 2nd, 2026 they will span at least the underlying data quality, accuracy, fairness, robustness, explainability, transparency, safety and cybersecurity of AI systems. Enormous fines are on the line in case of non-compliance, with up to 15 million euros or 3 percent of the offender’s total worldwide annual turnover awaiting offenders.
“Conformity assessments the easy way – automated, audit-ready, across time.”
At spotixx, in our new transfAIr project, we develop a software solution that allows financial institutions to implement the complex EU conformity assessments the easy way. Backed by a cutting-edge academia and industry cooperation together with Goethe University Frankfurt, our software toolkit will enable a straightforward procedure to assess the compliance of high-risk AI models: automated, audit-ready and scalable across time. As a result, spotixx is not only committed to state-of-the-art AI solutions for financial services. With transfAIr, we are helping financial institutions to run fair and ethically sound AI systems. Our mission is the responsible use of AI today – for safer and better financial services tomorrow.
Do you want to collaborate in our joint work towards responsible AI? Are you interested in automated and straightforward conformity assessments with the new EU legislation? Do you need support in building responsible and fair AI models? Feel free to reach out to us and add to our research project transfAIr!
Use our message form here or click on Lion’s image to find him on LinkedIn.
Literature
Blokland, T. and Vief, R. 2021. Making Sense of Segregation in a Well-Connected City: The Case of Berlin. In: van Ham, M., Tiit, T. Ubarevičienė, R. and Janssen, H. (eds.), Urban Socio-Economic Segregation and Income Inequality. Springer.